
Insightful Exploration of Large Language Models: The document begins by defining LLMs and highlighting their potential applications across various industries. It emphasizes the significant opportunities that LLMs offer in terms of natural language processing, content generation, and automation. By outlining the general opportunities and specific IT security benefits of LLMs, the document effectively sets the stage for a comprehensive discussion on the subject (Page 4).
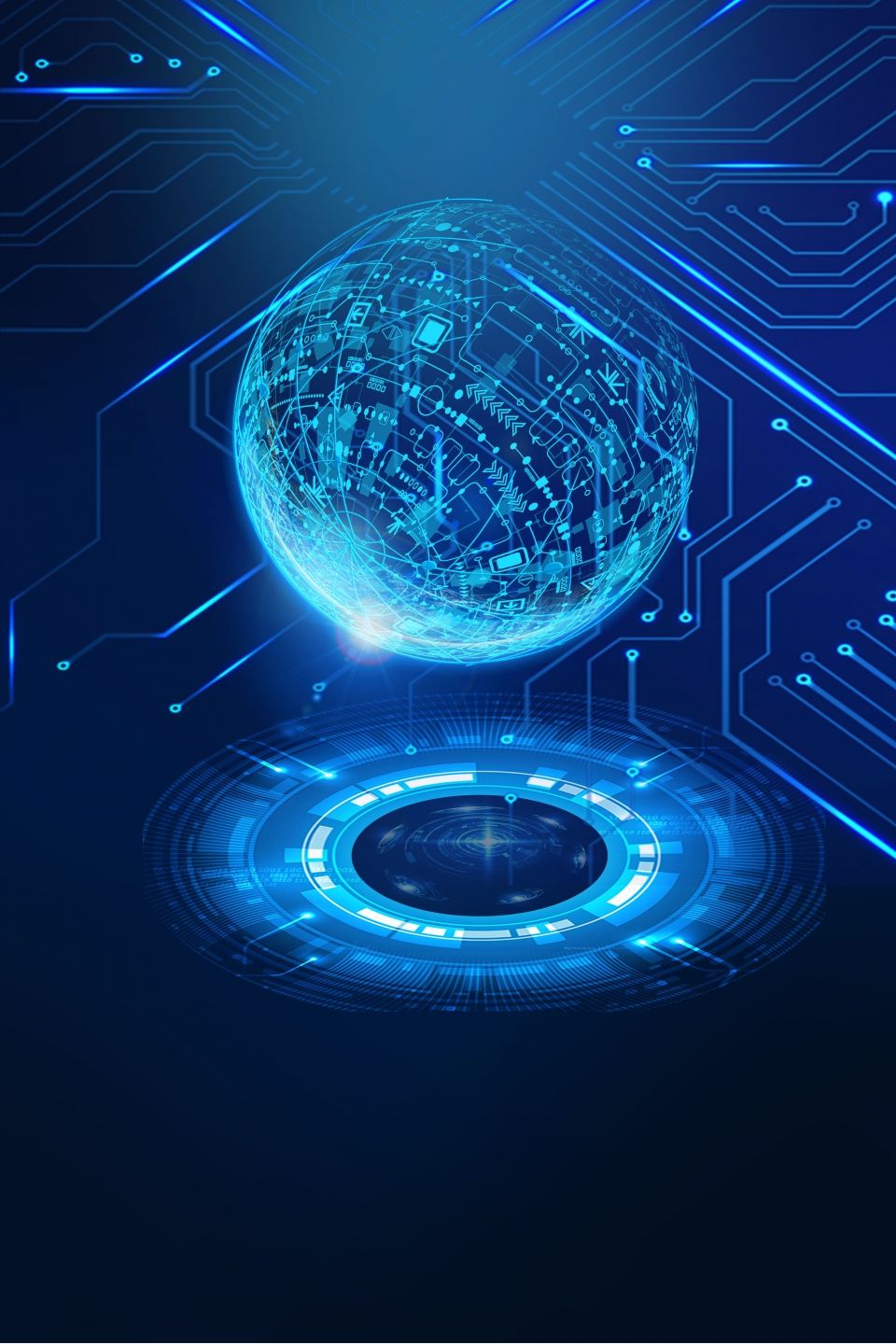
Addressing Risks and Challenges: One of the standout features of the document is its in-depth analysis of the risks associated with LLMs. It categorizes risks into proper use, misuse, and attacks, providing a structured approach to understanding and mitigating potential threats. The document acknowledges the challenges posed by the generation of malicious code using LLMs, highlighting the need for improved detection methods to combat such threats effectively (Pages 12 & 23).
Comprehensive Countermeasures: To mitigate the risks associated with LLMs, the document offers a range of countermeasures that organizations and users can implement. It stresses the importance of raising awareness among users about the security aspects of LLMs, conducting thorough testing before deployment, and handling sensitive data with caution. By emphasizing the need for extensive testing, red teaming, and data protection measures, the document provides practical guidance for enhancing the security posture of LLM-based systems (Pages 28 & 20).
Practical Recommendations for Secure Usage: The document goes beyond theoretical discussions and offers practical recommendations for ensuring the secure usage of LLMs. It suggests that users should be informed about the risks of data leakage, output quality issues, and potential misuse scenarios. Moreover, it advocates for the implementation of role-based access control mechanisms to limit the dissemination of sensitive information generated by LLMs. By emphasizing the importance of user awareness and data protection, the document aligns with best practices in cybersecurity (Page 28).
Testing and Evaluation Framework: A notable aspect of the document is its emphasis on conducting comprehensive tests to evaluate the performance and security of LLMs. It recommends the use of appropriate testing methods and benchmarks to cover edge cases and uncover vulnerabilities. The inclusion of red teaming as a testing methodology underscores the proactive approach advocated by the document in identifying and addressing potential weaknesses in LLM-based systems. By highlighting the importance of continuous testing and improvement, the document promotes a culture of security awareness and resilience (Page 20).
Conclusion: In conclusion, the document “Generative AI Models” by the Federal Office for Information Security serves as a valuable resource for understanding the opportunities and risks associated with Large Language Models. Its comprehensive coverage of the subject, practical recommendations for secure usage, and emphasis on testing and evaluation make it a relevant and insightful guide for organizations and individuals working with LLMs. By addressing the evolving security challenges posed by generative AI models, the document contributes to the ongoing dialogue on responsible AI deployment and cybersecurity best practices.
Related Articles
Related
Artificial Intelligence Index Report 2023
Shift in AI Development Leadership Historically, academia led in releasing significant machine learning models. Since 2014, the industry has taken the helm, producing 32 major models in 2022 alone, compared to just three from academia. This shift is largely due to the...
Generative AI is poised to dramatically enhance productivity
Generative AI is poised to dramatically enhance productivity, potentially adding up to $4.4 trillion annually to the global economy. This figure surpasses the entire GDP of the United Kingdom in 2021, highlighting the immense economic potential of this technology....
AIdeas from the Ground Up: Revolutionizing Innovation with Artificial Intelligence
How Companies Can Foster and Organize Idea Sharing from the Bottom Up In the ever-evolving landscape of business, companies that embrace innovation are the ones that lead. Encouraging idea sharing from the bottom up not only fosters a culture of engagement and...